Listen to this article
TL;DR: We built a Firebolt-powered support chatbot using retrieval-augmented generation (RAG). Our chatbot delivers accurate, domain-specific answers using Firebolt’s low-latency data warehouse.
Key Takeaways:
- Firebolt as a vector database: Store and retrieve embeddings at scale while leveraging Firebolt’s low-latency query engine.
- Fast vector search: Firebolt’s vector similarity search capabilities ensure low-latency responses — critical for real-time AI applications.
- Hands-on implementation: Our open-source GitHub repository lets you build your own Firebolt-powered chatbot with your data, or with the example dataset provided there.
Introduction
We’re building a Firebolt support chatbot to answer users' questions instantly, eliminating the need to search through documentation or wait for support responses. By leveraging retrieval-augmented generation (RAG) with Firebolt as the vector database, the chatbot efficiently retrieves relevant information, streamlining the user experience. Now, users can get accurate, data-driven answers without the friction of manual searches or delays. This blog will describe in detail how we implemented the chatbot. Additionally, we will provide a GitHub repository with code for building your own Firebolt-powered chatbot using your own data source! To get you started, the repository includes an example dataset as well.
Why Did We Use RAG?
Before we talk about why we used RAG, it's important to understand “What is RAG?”. RAG is a technique for improving the accuracy of large language models (LLMs) and reducing the number of hallucinations. To use RAG, we must first have a data source that contains documents. Our data source for the Firebolt chatbot includes Firebolt 2.0 documentation, some internal design documents, and documentation for Firebolt’s development tools. We were careful about not revealing internal Firebolt information to customers. The internal documents will only be used if the user of the chatbot is a Firebolt employee, and we have a mechanism to ensure that.
When we have the data source, we split the documents into smaller pieces of text called chunks. Next, the chunks are sent to an embedding model. An embedding model converts text into vectors called embeddings. Those embeddings capture the semantic meaning of the text. Next, the embeddings of the chunks are stored in a database. Many RAG systems use vector databases to store embeddings. Vector databases are specifically designed to store vectors and allow users to retrieve them quickly. An example is Pinecone. We used Firebolt as a vector database!
When the user asks the chatbot a question, the question goes to the embedding model, which converts the question into an embedding. Now, we need to find the embeddings that are similar to the embedding of the question. That requires vector similarity search, which Firebolt supports efficiently. Vector similarity search means we go to the database and retrieve the top k embeddings that are the most similar to the user’s question. The similarity is calculated using one of Firebolt’s vector similarity metrics. Finally, we get the chunks that correspond to those top k embeddings. Those are the top k most similar chunks to the question. We pass those chunks to the LLM, along with the user question and the prompt. The LLM uses the chunks as context to form its response to the question. The diagram below illustrates the RAG workflow.
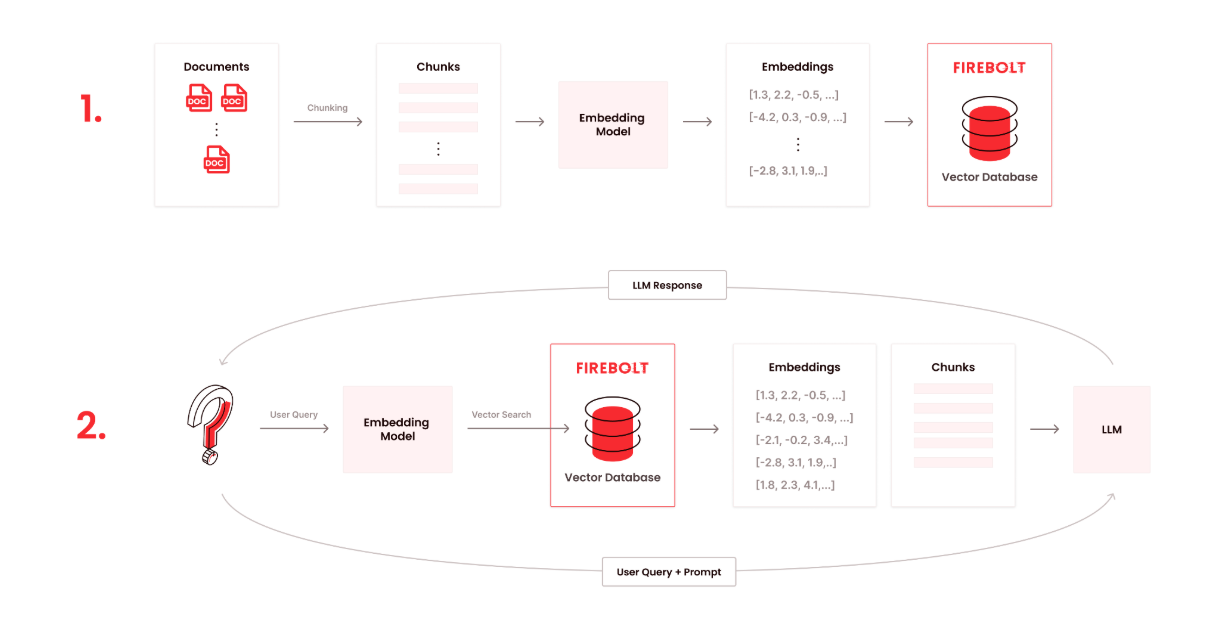
So, why RAG? Most LLMs have general knowledge across many domains, and they are not optimized for the specific domain of answering Firebolt support questions. With RAG, we can give the LLM the Firebolt-specific information that it needs to answer Firebolt support questions. Without RAG, the LLM could hallucinate because the answer to the user’s question may not be in its training data.
How We Implemented RAG
Now that we went over the fundamentals of RAG, let’s talk about how we implemented our own RAG system for Firebolt. Here, we will go through every step of our RAG architecture in detail.
The Data Source
As we previously mentioned, the data source for the Firebolt chatbot includes the Firebolt 2.0 documentation, internal design documents, and the documentation for Firebolt’s development tools. We will continuously keep adding documents to the data source in order to make it complete and comprehensive.
We had challenges with parsing these documents into text. This is because the documents had many different file types (such as HTML, Markdown, and Word), and they contained tables. So, our RAG system’s ability to parse documents is not 100% perfect.
Chunking
There are a variety of strategies that can be used to chunk documents for RAG. Here are the chunking strategies that our RAG system supports:
- Making every sentence its own chunk
- Making every paragraph its own chunk
- Making each chunk a fixed number of words (for example, 100 words).
- Recursive character text splitting
- Chunking every n sentences with a sliding window
- Semantic chunking
- This involves embedding every sentence in the document, comparing the similarity of those embeddings, and grouping the sentences with similar embeddings into the same chunk. Semantic chunking ensures that the semantic meaning of the text is preserved.
The chunking strategy we are currently using in our RAG system is semantic chunking. We concluded that semantic chunking was the best at keeping the chunks coherent. Other chunking strategies might not keep paragraphs, sentences, lists, or tables together in the same chunk. However, semantic chunking isn’t perfect either: it occasionally had those problems too. Also, semantic chunking might take longer than the other chunking strategies, and it often produces very long chunks.
The best chunking strategy depends heavily on the input data. For example, suppose we have documents that simply contain paragraphs of text and do not contain code, lists, or tables. In that case, we could chunk by every n sentences with a sliding window. For that type of document, the sentence tokenizer would correctly detect where sentences start and end. Also, the overlap between chunks would preserve context. However, our data source contains a lot of code, lists, and tables, so the idea of “sentences” does not apply. Chunking by every n sentences with a sliding window would not create semantically meaningful chunks for our data source.
The Embedding Model
We used nomic-embed-text as the embedding model. It creates vectors with 768 dimensions. We chose nomic-embed-text because it has a large context window, it is high-performing on long-context tasks, and it is open-source. nomic-embed-text has a context window of 8,192 tokens, which is helpful because semantic chunking often creates large chunks.
Firebolt as a Vector Database
We used Firebolt as the vector database. “Vector database” usually refers to specialized systems like Pinecone that only support vectors. However, Firebolt supports several vector similarity and vector distance metrics, and we used one of them to perform vector similarity search. In that way, it serves as the vector database for our RAG system. The cool thing about Firebolt is that you can use it as a vector database, but also as a general SQL database!
To create the table that stores the embeddings, we wrote a Python function that uses the Firebolt SDK to run a CREATE TABLE statement in Firebolt. The code below is a simplified version of that CREATE TABLE statement. table_name is a placeholder for the actual name of the table.
CREATE TABLE IF NOT EXISTS table_name(
document_id TEXT NOT NULL,
document_name TEXT NOT NULL,
document_version TEXT NOT NULL,
repo_name TEXT NOT NULL,
chunk_content TEXT NOT NULL,
chunk_id TEXT NOT NULL,
chunking_strategy TEXT NOT NULL,
embedding ARRAY(FLOAT NOT NULL) NOT NULL,
embedding_model TEXT NOT NULL,
date_generated DATE NOT NULL,
internal_only BOOLEAN NOT NULL
) PRIMARY INDEX date_generated, document_id
The following table contains descriptions of each column in the Firebolt table:
Here’s how this table schema simplifies management. For each chunk, the document ID and document name are stored. That way, we know which document that chunk is from. We also have the document version and the date that each row was ingested. That makes it easy to manage updates to documents. If we update the document later and ingest the updated document, the date generated and the version will be different, so we’ll know that this is the most recent version of the document. Therefore, if there are updates to the data source and the old documents become outdated, we don’t have to delete the old documents from the table. With this table schema, it will be very easy to implement a mechanism for retrieving the latest version of a document. Furthermore, the internal_only column indicates whether the document is an internal Firebolt document or a user-facing one. That way, if the chatbot user is a customer, we can filter out the internal documents, and if the user is a Firebolt employee, we can keep them. Also, the chunking_strategy column allows us to filter by chunking strategy so we can compare different chunking strategies. That allows for easy improvements to the chatbot.
Loading the Data
To load the documents for the data source into Firebolt, we first had to prepare the data. This involved the following steps:
- Getting the documents from the user’s local GitHub repository
- Parsing the documents into text
- Obtaining the version of each document
- Chunking each document
- Embedding the chunks
- Obtaining all the other information in the Firebolt table’s schema during the steps above
After that, we populated the Firebolt table. The generate_embeddings_and_populate_table() function first performs all of the data preparation steps by calling various helper functions. Then, it populates the Firebolt table by calling the populate_table() function. populate_table() inserts the data into the table in batches of many rows at a time. The user can specify the batch size using the batch_size parameter of generate_embeddings_and_populate_table(). That method of ingesting data is much faster than inserting one row at a time, which creates a better user experience. Below are the method signatures and shortened versions of the docstrings for each of those functions:
def generate_embeddings_and_populate_table(
repo_dict: dict,
chunking_strategies: list[ChunkingStrategy],
batch_size: int = 100,
rcts_chunk_size: int = 600,
rcts_chunk_overlap: int = 125,
num_words_per_chunk: int = 100,
num_sentences_per_chunk: int = 3
) -> None:
"""
Prepares the data and populates the table.
Parameters:
- repo_dict - dictionary containing file paths to repositories,
names of the main branches, and whether the repos contain
internal docs
- chunking_strategies: all chunking strategies to use
- batch_size: Number of rows to insert into the Firebolt table at
a time. (e.g.: batch_size = 100 will insert your data into the
table in batches of 100 rows at a time).
- rcts_chunk_size, rcts_chunk_overlap: Chunk size and overlap for
recursive character text splitting
- num_words_per_chunk: number of words per chunk, when chunking
using a fixed number of words
- num_sentences_per_chunk: number of sentences per chunk, when
chunking by sentences with a sliding window.
Returns: nothing
"""
def populate_table(
data_dict: dict,
table_name: str,
batch_size: int
) -> None:
"""
Populates the Firebolt table, inserting batch_size rows at a time.
Parameters:
- data_dict: dictionary containing the data to populate the table
with.
- table_name: name of the Firebolt table
- batch_size: number of rows to insert at a time
Returns: nothing
"""
Vector Search
To perform vector search, we wrote a Python function that runs a SQL query on Firebolt using its Python SDK. The code below is the function signature and a shortened version of the docstring.
def vector_search(
question: str,
k: int,
chunking_strategy: str,
similarity_metric: VectorSimilarityMetric,
is_customer: bool = True
) -> list[list]:
"""
Performs vector search and returns the top k most similar rows to the user's question.
Parameters:
- question: the user's question
- k: how many chunks to return
- chunking_strategy: chunking strategy of the chunks you want to
retrieve.
- similarity_metric: similarity metric to use for vector search
- is_customer: whether the chatbot user is a customer or an
employee
Returns: the k most similar rows.
"""
Firebolt supports several vector similarity and vector distance metrics. We used cosine similarity for our RAG system. However, in the open-source GitHub repository, we have provided the option to use any Firebolt vector similarity or distance metric, so that users can explore Firebolt’s vector capabilities. Cosine similarity is commonly used for semantic search and other text analysis applications. Cosine similarity only depends on the directions of the two vectors we are comparing, and not their magnitudes. So, it’s ideal for finding the semantic similarity between two pieces of text, regardless of the lengths of the texts. That means that, for our use case, cosine similarity would return the most relevant chunks to the query.
Suppose we have a user query, and we want to find the most relevant chunks. The chunks in our vector database almost always contain more text than the user queries. Therefore, if we have a chunk that’s very semantically related to the query, the embedding of the chunk and the embedding of the query may have very different magnitudes. Metrics that compare vectors by magnitude would indicate that the chunk and the query are far apart, even though they are very semantically similar. So, that chunk may not appear in the top k most relevant chunks returned by vector search. Comparing the directions of the two vectors, however, measures whether two documents are contextually related. Therefore, our desired chunk, and the other chunks that are contextually related to the query, would appear in the top k chunks.
The caller of the vector_search() function can choose which similarity metric to use. Next, for the example SQL query, assume the following:
- The desired chunking strategy is semantic chunking
- k = 10
- We want to use cosine similarity
- The user is a customer
- The embedding model we are using to embed the query is nomic-embed-text. (This is not a function parameter, but we filter by the embedding model inside the query because the Firebolt table may contain embeddings from different models).
With those assumptions, the code below is an example Firebolt SQL query that performs vector search. In the code, question_vector is a placeholder for the embedding of the user’s question. table_name is a placeholder for the name of the Firebolt table that serves as the vector database.
SELECT chunk_content,
vector_cosine_similarity(question_vector, embedding),
document_name
FROM table_name
WHERE chunking_strategy = 'Semantic chunking'
AND internal_only = FALSE
AND embedding_model = 'nomic_embed_text'
ORDER BY vector_cosine_similarity(question_vector, embedding) DESC
LIMIT 10;
For this query, note that the Python function sets up the ORDER BY clause to use ascending or descending order depending on whether a vector similarity metric or a vector distance metric is used. For vector similarity metrics, the higher the value of the metric, the more similar the two vectors are. For vector distance metrics, the higher the value of the metric, the more different the two vectors are. Therefore, if we are using a similarity metric, we order the results in descending order (DESC) to get the top k most similar chunks. If we are using a distance metric, we order the results in ascending order (ASC) to get the top k most similar chunks. Firebolt’s vector similarity functions are VECTOR_COSINE_SIMILARITY() and VECTOR_INNER_PRODUCT(). Our vector distance functions are VECTOR_COSINE_DISTANCE(), VECTOR_EUCLIDEAN_DISTANCE(), VECTOR_MANHATTAN_DISTANCE(), and VECTOR_SQUARED_EUCLIDEAN_DISTANCE().
Vector databases index vectors to allow for faster similarity search. Firebolt does not support that type of indexing yet. However, when we use Firebolt as a vector database for RAG, the number of rows in the Firebolt table will always be small (for example, a million rows). Nobody uses a very large number of rows (for example, a billion) for RAG. A million chunks is a lot of text, so we can fit a lot of knowledge for a domain-specific chatbot into the Firebolt table. So, if we have a small number of rows, and then we filter, we don’t need to do special indexing the way that vector databases do, and our similarity search is still fast!
Firebolt’s Similarity Search Performance
One thing that’s unique about Firebolt is its high performance. To demonstrate that, we will show how long it takes to perform similarity search on our Firebolt table, as we vary k, the number of chunks returned.
Our table has 1,525 rows, and all the embeddings were created by nomic-embed-text, so they have 768 dimensions. For each k-value, we ran our SQL query for similarity search for 300 iterations. We measured the time that each of these 300 iterations took. For all the similarity search queries, the question we asked the chatbot was “How are primary indexes implemented in Firebolt?” and the similarity metric used was cosine similarity. Next, for each k-value, we calculated the median and the 95th percentile of the similarity search time. The results of our measurements are in the graph below.

For every k under 1000, the median and the 95th percentile are subsecond! For k = 1000, the median vector search time is almost a second, and the 95th percentile is more than a second. However, similarity search for RAG will never return 1000 chunks. In fact, it should not return more than 20 or 30 chunks for several reasons.
First of all, LLMs have a limited context window. The context window is how much text the LLM can remember at once, including both the input prompt and the output text that it generates. Thus, if we give the LLM more information than can fit in the context window, it will not be able to remember all that information. Most likely, a very high number of chunks like 1000 will not be able to fit in the context window.
Furthermore, vector similarity search orders the chunks by how similar they are to the question. When we return a very large number of chunks, such as 100 or 1000, the results near the top of the list will have a high similarity to the question, but the results near the bottom of the list will most likely have a very low similarity to the question. Thus, the chunks at the bottom of the list will be irrelevant to the question and unnecessary.
Finally, the more text we pass to the LLM, the longer it will take for the LLM to process that text. So, passing hundreds of chunks both slows down the LLM’s performance and gives the LLM irrelevant chunks. For these reasons, it is not beneficial to RAG systems to use a very large number of chunks. Therefore, Firebolt’s vector similarity search performance for k = 1000 chunks is irrelevant. Also, note that 500 chunks are still too many for RAG, and 95% of the time, Firebolt still takes much less than a second to perform similarity search for k = 500. Because RAG systems should use a small number of chunks, Firebolt still efficiently supports vector similarity search for RAG, with subsecond performance.
The LLM
We used Llama3.1 as the LLM for the chatbot. The advantage of Llama models is that they are open source. Some services for accessing AI models, like OpenAI, require a paid subscription, which increases the monetary cost of this project. With Llama, we don’t have that concern anymore.
Below is the method signature and a shortened docstring of the Python function that gives the LLM a prompt that includes the user’s question and the vector search results. That function returns the LLM’s response. For the entire function, please see the run_chatbot() function in this file.
def run_chatbot(
user_question: str,
session_id: str,
chat_history_dir: str = "chat_history",
chunking_strategy: str = "Semantic chunking",
k: int = 10,
similarity_metric: VectorSimilarityMetric =
VectorSimilarityMetric.COSINE_SIMILARITY,
print_vector_search: bool = False,
is_customer: bool = True
) -> str:
"""
Runs the chatbot once.
Parameters:
- user_question: the question the chatbot user asked
- chat_history_dir: the directory to store the chat history files
in
- chunking_strategy: the vector search will only retrieve chunks
that have this chunking strategy
- k: how many chunks to retrieve
- similarity_metric: similarity metric to use for vector search
- print_vector_search: Whether to print the vector search results
- is_customer: whether the chatbot user is a customer or
an employee
- session_id: the unique ID of this chatbot session
Returns: the bot's response to the question
"""
A key consideration was the size of the LLM’s context window. LLMs split the input text they are given into smaller pieces called tokens, and the context window is measured by the number of tokens that it can fit. A large context window would allow the LLM to remember more of the previous conversation with the user. That would create a better user experience, and the LLM would have more information that it may need to answer future questions. On the other hand, a large context window means that the LLM will be slower in generating its response, which would create a negative user experience. To balance these two factors, we chose to use a context window of 5,000 tokens, which is about 3,750 words.
Extensions
We have much potential for future work and extensions to the Firebolt chatbot. There was research in Firebolt about a code copilot. This is an LLM that would help users fix errors in their Firebolt SQL code when the SQL doesn’t compile. Users would feed in the incorrect Firebolt SQL code, and the LLM would generate the correct code.
Furthermore, we can implement a mechanism to filter the similarity search results by the most recent version of a given document, and the most recent version of Firebolt. For the former, we can filter by the date_generated column and/or the document_version column in the SQL query for similarity search, in order to get the most recent version of each document. For the latter, we have documentation for different versions of Firebolt. Every time we release a version of Firebolt, we will update the data source with the documentation for the new version. We can add a column to the Firebolt table that stores the Firebolt version that each chunk pertains to. Next, inside the SQL query that performs vector search, we could filter by Firebolt version, obtaining only the documentation for the most recent version. This enhanced filtering will speed up the vector search operation even more!
Conclusion
Our Firebolt support chatbot is powered by a RAG system that is accurate at answering Firebolt-specific questions, fast, has a low monetary cost, and carefully protects sensitive data. We have put careful consideration into every component of our RAG system, from the chunking strategy and the context window, to data privacy needs. The result is an accurate, secure, and domain-specific chatbot that both customers and employees can trust.
One of the pain points of RAG is that vector similarity search is computationally expensive, and therefore it is often slow. However, vector similarity search is very fast in Firebolt, which we have shown with benchmarks. Therefore, Firebolt is capable of playing a crucial role in building efficient RAG systems!
Thanks to our vector search capabilities, you can use Firebolt similarly to any other vector database. Furthermore, Firebolt allows you to store your vector data and your other data together in one platform. Specialized vector databases such as Pinecone or Chroma only support vectors, and you would need to use another service to store other kinds of data. Thus, with Firebolt, you can use fewer services. We address vector capabilities and your analytical needs in one database!
Finally, this GitHub repository contains code and instructions for running your own Firebolt-powered chatbot on your local machine! You may use your own data source or the example dataset in the repository.
Acknowledgements
I’d like to give credit to everyone who helped me make the Firebolt support chatbot possible. Thank you to Hiren Patel, Pascal Schulze, Max Reinsel, and Richard Gutierrez.